Research Interests
1. Electrified mobility: advanced modeling and control for battery and powertrain;
2. Machine learning-based diagnostics and prognostics
3. Autonomous vehicles
4. Energy storage systems
​
Autonomous driving
The future of mobility: Autonomous, Connected, Electric, Shared.
​
Sensing, localization, path planning, sensor fusion, AI-based auto driving
Efficient and Fast Pseudo Point Cloud Generation from Stereo Depth Estimation
​
The proposed work presents an accurate point cloud projection from camera-based depth estimation. The depth generation approach outperforms other existing approaches on the KITTI benchmark. The encoder network is modified to feed stereo images as an input feature. At the same time, this method generates the point cloud in real-time since the approach adopted U-Net architecture instead of other heavy and complex depth networks. This proposed method will radicalize the robotic society to use vision-based sensors instead of laser-based since LiDARs are very expensive.

High-Definition Map for Autonomous Driving
​
High-Definition (HD) map is one of the critical components of the autonomous driving system. HD maps are known for their high precision and rich geometric and semantic information about the autonomous vehicles’ surrounding environment. They contain all critical static properties of the environment, such as road boundaries, buildings, traffic lights, road markings, road furniture, and traffic rules which are necessary for autonomous driving.

Machine learning based power management of PHEV
​
Motivations : the existing power management systems for PHEVs are developed base don pre-sampled driving cycles, while the real-world driving cycles can vary greatly depending on the traffic conditions, and therefore be much different comparing to the pre-sampled ones.
Method: To reduce the energy consumption in real-world driving cycles, the real-time traffic data is fed into an machine learning algorithm to extract the optimal control policy given the real-time traffic conditions.
Results: a 7.12% reduction in fuel consumption is achieved
​

Machine learning based state estimation, diagnostics and prognostics
​
Motivations : advanced battery management systems must take into account the internal status of lithium ion batteries in order to ensure safe operation and to prolong the battery useful life.
​
Method: Neural network based internal status estimation, such as, temperature, stress, lithium plating, SOC, etc… And also prediction (prognostics) of thermal runaway, or severe side reactions during operation.
​
Results: high prediction accuracy of internal status based on machine learning method. High accurate prediction of severe side reactions.
​
​
Multiphysics modeling, optimal design and health conscious fast charging
​
1. Multiphysics modeling based on experimental degradation analysis
Motivation: How do Li-ion batteries degrade over long-term cycling (degradation analysis)
​
​
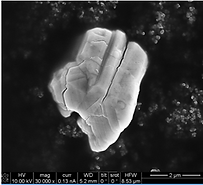
SEM examination of cathode fracture
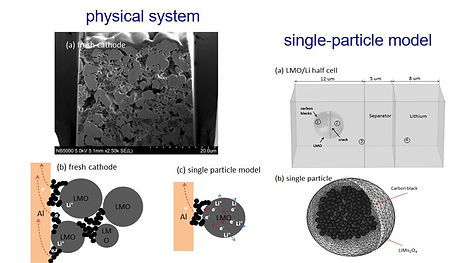
2. Battery sensing
Motivations: Knowing the internal states is extremely important
​
​
​

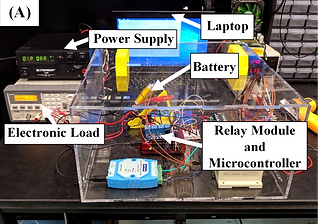
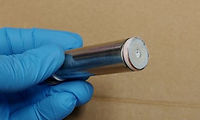
Internal thermal monitoring
3. Health conscious charging of battery packs
Motivations: How should we control Li-ion batteries to achieve longer cycle life (optimal control)
Method: Physics based modeling, optimal design, and advanced control
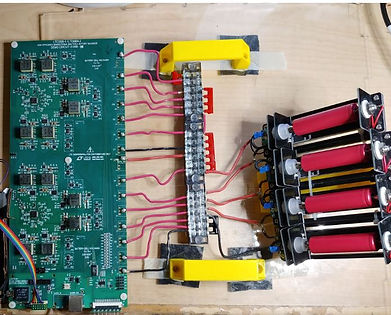
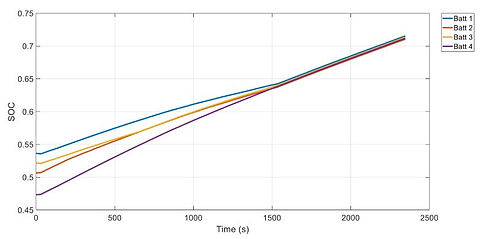